Zach del Rosario '14
he / him / his
Assistant Professor of Engineering
Active
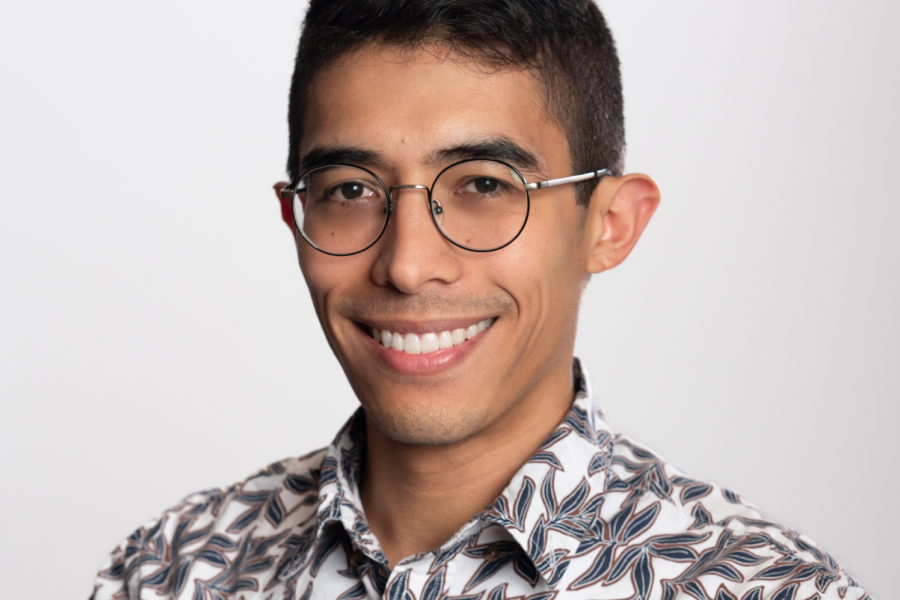
education
- PhD, Aerospace Engineering, Stanford University
- MS, Aerospace Engineering, Stanford University
- BS, Mechanical Engineering, Olin College
awards
- Association of American Colleges and Universities (AAC&U) Patricia Cross Future Leaders Award (2020)
- Stanford Vice Provost for Graduate Education (VPGE) Diversifying Academia, Recruiting Excellence (DARE) Fellowship (2018)
- National Science Foundation (NSF) Graduate Research Fellowship Program (GRFP) (2015)
research
- Tools:
- Uncertainty Quantification
- Statistics
- Data Science
- Applications:
- Structural Safety
- Materials Informatics
- Education
website
http://www.zdelrosario.com/Zach graduated from Olin College in 2014 with the desire to become a professional educator. Six years later he earned a PhD in Aerospace Engineering and returned to Olin to start his faculty career.
In his research, Zach helps scientists and engineers make decisions under uncertainty. In his PhD work he discovered long-standing probability errors in aircraft design that expose travelers to risk, and developed alternative design criteria with mathematically-provable safety guarantees. Zach also consults with scientists from other disciplines: He has worked with material scientists to use machine learning for accelerated materials R&D.
Presently, Zach is developing a Grammar of Model Analysis to support the teaching and communication of model analysis under uncertainty.
In his teaching, Zach strives to cultivate an inclusive and supportive class environment. He works to help all students become self-directed learners, emphasizes communication alongside analysis, and encourages students to embrace uncertainty. Zach's classes tend to emphasize student-directed work and open discussion.