Gretchen Rice ’20 believes working beside a robot should be no different than working with a human being. “You can't really implement robots into the world if humans aren’t comfortable interacting with them or humans don't understand them,” Rice says.
As part of her Research Experience for Undergraduates (RUE), Rice spent this summer at Harvey Mudd College in Claremont, California, studying human-robot interactions in the workplace.
She worked with Seth Isaacson, an undergraduate at the school, and James C. Boerkoel Jr, an associate professor of computer science there, to develop a new assessment tool for measuring the ease or difficulty humans experience while collaborating with robots.
"We believe our ongoing explorations will be key in understanding, designing, and planning more fluent human-robot interactions.” - Gretchen Rice ’20
Rice (center) with colleagues at Harvey Mudd College in Claremont, California. Rice is studying human-robot interactions in the workplace.
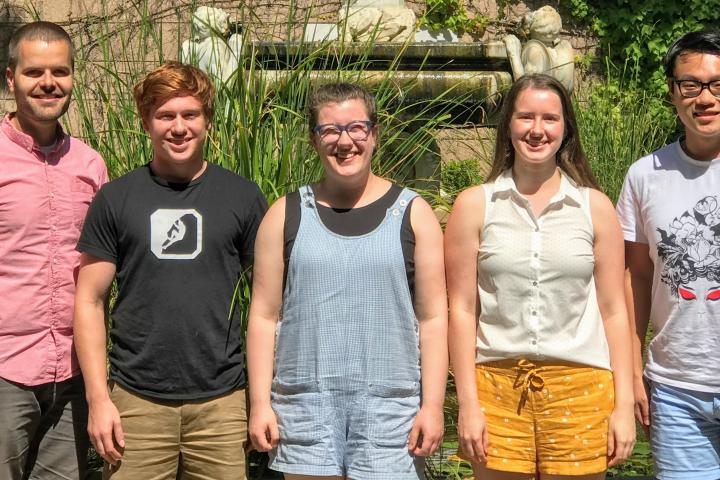
Rice is now a published scientific author. In September, she, Isaacson and Boerkoel published a description of their assessment tool, which they named the Multi-Agent Daisy Temporal Network (MAD-TN). The paper was accepted to the 2019 AAAI Fall Symposium on Artificial Intelligence for Human-Robot Interaction, which they will be attending in DC this November.
To develop MAD-TN, Rice and her colleagues asked human participants to work side by side with Sawyer, a robotic arm built in the United States. Sawyer and its human coworker had to pack a medium-sized plastic container together.
The job was a lot harder than it sounds. Three smaller boxes needed to go inside the container. One of those small boxes needed to be packed with several toys. And it all had to be done as efficiently and quickly as possible so that while the human might be retrieving one of the toys, Sawyer might be readying one of the smaller boxes.
Typically, scientists use a metric called fluency to measure how well humans and robots are cooperating. Fluency gets broken down into such criteria as the amount of time the human or robot is idle or the amount of time both are active at the same time. The goal is to optimize fluency to maximize efficiency and productivity.
As they observed their participants and Sawyer carrying out their job, Rice and her team realized that the current method of measuring fluency wasn’t adequate to truly evaluate how well robot and human work together.
As a result, the researchers developed two new additional criteria for assessing fluency. One is called “resource delay.” In Rice’s experiment, a box is an example of a resource. Resource delay assesses how well they share it by measuring the time between when the human finishes with the box and the robot starts using it to carry out its task.
The second metric Rice’s team developed is “concurrent inactivity.” It measures the worst-case scenario when humans and robots work together — the amount of time both are idle.
The existing fluency assessment method plus resource delay and concurrent inactivity are the key components of MAD-TN.
Rice, Isaacson and Boerkoel plan to continue to refine MAD-TN. They believe ultimately it will help researchers design robots that humans might even enjoy working with. As they wrote in their paper, “We believe our ongoing explorations will be key in understanding, designing, and planning more fluent human-robot interactions.”